Dr. Chen Qin |
Tue 20 Oct 2020, 13:00 - 14:00 |
Online Teams |
If you have a question about this talk, please contact: Mehrdad Yaghoobi Vaighan (myvaigha)
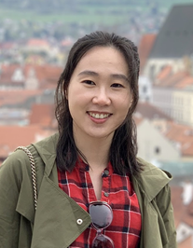
Abstract: Recent advances in machine learning have shown great potentials in improving the entire medical imaging workflow, from image acquisition and reconstruction to disease diagnosis. In this talk, I will mainly focus on machine learning for Magnetic Resonance (MR) image reconstruction and analysis. Firstly, I will introduce my recent study on accelerated dynamic MR image reconstruction from highly undersampled k-space data, and will discuss a few model-based deep learning methods with an application on cardiac cine MRI reconstruction. Secondly, I will present works on learning with limited supervisions for medical image analysis, including weakly-supervised image segmentation, self-supervised/unsupervised mono-/multi-modal image registration and biomechanics-informed neural network for myocardial motion tracking. Lastly, I will briefly discuss about some applications on the integration of MRI reconstruction and analysis, which could potentially facilitate the fast and specialised MR imaging and analysis.
Bio: Dr. Chen Qin is a Lecturer in Computer Vision and Machine Learning at Electronics and Electrical Engineering, The University of Edinburgh. She is also an Honorary Research Fellow at Imperial College London. Before that, she worked as a Research Associate at Department of Computing, Imperial College London. She also obtained her Ph.D. in Computing Research from Imperial College London. Her research is at the interdisciplinary field of artificial intelligence and medical imaging, aiming to improve the entire medical imaging/radiology workflow with significant impact for clinical use via machine intelligence. Her current research mainly focuses on the development of machine learning algorithms for magnetic resonance image reconstruction and analysis, including dynamic MR image reconstruction, medical image registration and segmentation.