Agisilaos Chartsias |
Thu 13 Sep 2018, 13:00 - 13:30 |
AGB Seminar Room AGB Building, King’s Buildings, EH9 3JL |
If you have a question about this talk, please contact: Ardimas Purwita (s1600157)
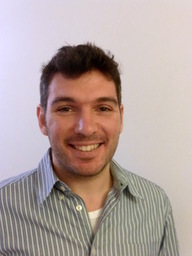
Agisilaos Chartsias is currently working towards the Ph.D. degree in the Institute for Digital Communications.
Abstract: The success and generalisation of deep learning algorithms heavily depend on learning good feature representations. In medical imaging this entails representing anatomical information, as well as prop- erties related to the specific imaging setting. Anatomical information is required to perform further analysis, whereas imaging information is key to disentangle scanner variability and potential artefacts. The ability to factorise these would allow for training algorithms only on the relevant information according to the task. To date, such factori- sation has not been attempted. In this paper, we propose a methodol- ogy of latent space factorisation relying on the cycle-consistency principle. As an example application, we consider cardiac MR segmentation, where we separate information related to the myocardium from other features related to imaging and surrounding substructures. We demonstrate the proposed method’s utility in a semi-supervised set- ting: we use very few labelled images together with many unlabelled images to train a myocardium segmentation neural network. Specifically, we achieve comparable performance to fully supervised networks using a fraction of labelled images in experiments on ACDC and a dataset from Edinburgh Imaging Facility QMRI.
Biography: Agisilaos Chartsias received his diploma degree in Electronic Engineering and Computer Science from the Technical University of Crete, Greece, followed by a MSc in Artificial Intelligence from the University of Edinburgh. He is currently a PhD student in the University of Edinburgh under the supervision of Dr. Sotirios Tsaftaris working on deep learning for medical image analysis.